- “create responses that will form the ‘voice’ of future AI”;
- “provide feedback to teach AI models to become more helpful, accurate, and safe”;
- “write clear, concise, factually and grammatically correct responses”;
- “coach an AI model by assessing the quality of AI-generated writing, reviewing the work of fellow writing evaluators, and crafting original responses to prompts”.
But before they ever risk leading to a godlike superintelligence or devastating mass unemployment, they first need training. Instead of using these grandiloquent chatbots to automate us out of our livelihoods, tech companies are contracting us to help train their models.
As well as providing our model with ‘gold standard’ material, we help it attempt to avoid ‘hallucinating’ – telling lies
Without better language data, these language models simply cannot improve. Their world is our word.
Hold on. Aren’t these machines trained on billions and billions of words and sentences?
Well, for starters, the internet is finite. And so too is the sum of every word on every page of every book ever written. So what happens when the last pamphlet, papyrus and prolegomenon have been digitized and the model is still not perfect? What happens when we run out of words?
Note the word human. Large language models do little but produce prose, much of which is already being published on the internet.
So couldn’t we train these models on their own output (so-called synthetic data)? Our cyborg internet – co-authored by us and our word machines – could then swell ad infinitum. No such luck. Training our current large language models on their own output doesn’t work. “Indiscriminately learning from data produced by other models causes ‘model collapse’ – a degenerative process whereby, over time, models forget the true underlying data distribution,” write Ilia Shumailov and colleagues in Nature. In other words, they go off the rails and tend towards producing nonsense. Feeding something its own effluvia leads to atrophy. Who would have thought?
- Shumailov explained to me that each time a model is trained on synthetic data, it loses awareness of the long tail of “minority data” that it was originally trained on (rare words, unusual facts etc).
- The breadth of knowledge is eroded and replaced by only the most likely datapoints – LLMs are at their core sophisticated text-prediction machines.
- So when your original, digital data is already biased – very English language-heavy, largely US-centric, and full of unreliable forum posts – this bias will only be repeated.
- This is especially true as concerns spread that the much-vaunted models will stop being able to improve before they’ve ever become that useful.
- Leading startup investment firm Sequoia has shown that AI firms will need to fill a $500bn revenue gap by the end of this year to keep investors satisfied.
- The word machines might be hungry; the capital behind them also has an appetite.
But it’s not just a question of accumulating more original words. These companies need the sort of writing that the model will seek to emulate, not merely absorb.
That’s where human annotators come in.
In Fritz Lang’s classic 1927 film Metropolis, the ancient Canaanite deity Moloch is reincarnated as an insatiable industrial machine.
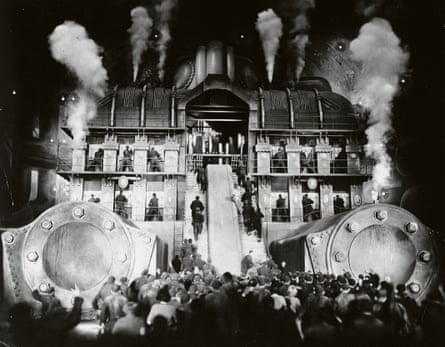
- It is like being paid to write in sand, to whisper secrets into a slab of butter.
- Even if our words could make a dent, we wouldn’t ever be able to recognize it.
- Large language models were supposed to automate writers’ jobs.
- The better they became through our work, the quicker our careers would decline.
- And so there I was, feeding our very own Moloch.
- It is like being paid to write in sand.
- Even if our words could make a dent, we wouldn’t ever be able to recognize it
Working for an AI company as a writer was therefore a little like being told you were going to be paid a visit by Dracula, and instead of running for the hills, you stayed in and laid the table. But our destroyer is generous, the pay sufficient to justify the alienation.
No comments:
Post a Comment