In an effort to make ChatGPT more reliable, the company has been focusing on cleaning up its dataset and removing examples where the model has had a preference for things that are false.
Tech Review Explains
Tech Review Explains
1
ChatGPT is everywhere. Here’s where it came from
But OpenAI’s breakout hit did not come out of nowhere. The chatbot is the most polished iteration to date in a line of large language models going back years. This is how we got here.
1980s–’90s: Recurrent Neural Networks
ChatGPT is a version of GPT-3, a large language model also developed by OpenAI. Language models are a type of neural network that has been trained on lots and lots of text. (Neural networks are software inspired by the way neurons in animal brains signal one another.) Because text is made up of sequences of letters and words of varying lengths, language models require a type of neural network that can make sense of that kind of data. Recurrent neural networks, invented in the 1980s, can handle sequences of words, but they are slow to train and can forget previous words in a sequence.
In 1997, computer scientists Sepp Hochreiter and Jürgen Schmidhuber fixed this by inventing LSTM (Long Short-Term Memory) networks, recurrent neural networks with special components that allowed past data in an input sequence to be retained for longer. LSTMs could handle strings of text several hundred words long, but their language skills were limited.
2017: Transformers
The breakthrough behind today’s generation of large language models came when a team of Google researchers invented transformers, a kind of neural network that can track where each word or phrase appears in a sequence. The meaning of words often depends on the meaning of other words that come before or after. By tracking this contextual information, transformers can handle longer strings of text and capture the meanings of words more accurately. For example, “hot dog” means very different things in the sentences “Hot dogs should be given plenty of water” and “Hot dogs should be eaten with mustard.”
2018–2019: GPT and GPT-2
OpenAI’s first two large language models came just a few months apart. The company wants to develop multi-skilled, general-purpose AI and believes that large language models are a key step toward that goal. GPT (short for Generative Pre-trained Transformer) planted a flag, beating state-of-the-art benchmarks for natural-language processing at the time.
GPT combined transformers with unsupervised learning, a way to train machine-learning models on data (in this case, lots and lots of text) that hasn’t been annotated beforehand. This lets the software figure out patterns in the data by itself, without having to be told what it’s looking at. Many previous successes in machine-learning had relied on supervised learning and annotated data, but labeling data by hand is slow work and thus limits the size of the data sets available for training.
But it was GPT-2 that created the bigger buzz. OpenAI claimed to be so concerned people would use GPT-2 “to generate deceptive, biased, or abusive language” that it would not be releasing the full model. How times change.
2020: GPT-3
GPT-2 was impressive, but OpenAI’s follow-up, GPT-3, made jaws drop. Its ability to generate human-like text was a big leap forward. GPT-3 can answer questions, summarize documents, generate stories in different styles, translate between English, French, Spanish, and Japanese, and more. Its mimicry is uncanny.
One of the most remarkable takeaways is that GPT-3’s gains came from supersizing existing techniques rather than inventing new ones. GPT-3 has 175 billion parameters (the values in a network that get adjusted during training), compared with GPT-2’s 1.5 billion. It was also trained on a lot more data.
But training on text taken from the internet brings new problems. GPT-3 soaked up much of the disinformation and prejudice it found online and reproduced it on demand. As OpenAI acknowledged: “Internet-trained models have internet-scale biases.”
December 2020: Toxic text and other problems
While OpenAI was wrestling with GPT-3’s biases, the rest of the tech world was facing a high-profile reckoning over the failure to curb toxic tendencies in AI. It’s no secret that large language models can spew out false—even hateful—text, but researchers have found that fixing the problem is not on the to-do list of most Big Tech firms. When Timnit Gebru, co-director of Google’s AI ethics team, coauthored a paper that highlighted the potential harms associated with large language models (including high computing costs), it was not welcomed by senior managers inside the company. In December 2020, Gebru was pushed out of her job.
January 2022: InstructGPT
OpenAI tried to reduce the amount of misinformation and offensive text that GPT-3 produced by using reinforcement learning to train a version of the model on the preferences of human testers. The result, InstructGPT, was better at following the instructions of people using it—known as “alignment” in AI jargon—and produced less offensive language, less misinformation, and fewer mistakes overall. In short, InstructGPT is less of an asshole—unless it’s asked to be one.
May–July 2022: OPT, BLOOM
A common criticism of large language models is that the cost of training them makes it hard for all but the richest labs to build one. This raises concerns that such powerful AI is being built by small corporate teams behind closed doors, without proper scrutiny and without the input of a wider research community. In response, a handful of collaborative projects have developed large language models and released them for free to any researcher who wants to study—and improve—the technology. Meta built and gave away OPT, a reconstruction of GPT-3. And Hugging Face led a consortium of around 1,000 volunteer researchers to build and release BLOOM.
December 2022: ChatGPT
Even OpenAI is blown away by how ChatGPT has been received. In the company’s first demo,
which it gave me the day before ChatGPT was launched online, it was
pitched as an incremental update to InstructGPT. Like that model,
ChatGPT was trained using reinforcement learning on feedback from human
testers who scored its performance as a fluid, accurate, and inoffensive
interlocutor. In effect, OpenAI trained GPT-3 to master the game of
conversation and invited everyone to come and play. Millions of us have
been playing ever since."
2 February 21, 2023
Correction: This story has been updated to reflect that Microsoft Bing is not built on ChatGPT, but similar AI language technology customized for search. We apologize for the error.
How OpenAI is trying to make ChatGPT safer and less biased
"It’s not just freaking out journalists (some of whom should really know better than to anthropomorphize and hype up a dumb chatbot’s ability to have feelings.) The startup has also gotten a lot of heat from conservatives in the US who claim its chatbot ChatGPT has a “woke” bias.
All this outrage is finally having an impact. Bing’s trippy content is generated by AI language technology similar to ChatGPT that Microsoft has customized specifically for online search. Last Friday, OpenAI issued a blog post aimed at clarifying how its chatbots should behave. It also released its guidelines on how ChatGPT should respond when prompted with things about US “culture wars.” The rules include not affiliating with political parties or judging one group as good or bad, for example.
I spoke to Sandhini Agarwal and Lama Ahmad, two AI policy researchers at OpenAI, about how the company is making ChatGPT safer and less nuts. The company refused to comment on its relationship with Microsoft, but they still had some interesting insights. Here’s what they had to say:
How to get better answers: In AI language model research, one of the biggest open questions is how to stop the models “hallucinating,” a polite term for making stuff up. ChatGPT has been used by millions of people for months, but we haven’t seen the kind of falsehoods and hallucinations that Bing has been generating.
That’s because OpenAI has used a technique in ChatGPT called reinforcement learning from human feedback, which improves the model’s answers based on feedback from users. The technique works by asking people to pick between a range of different outputs before ranking them in terms of various different criteria, like factualness and truthfulness. Some experts believe Microsoft might have skipped or rushed this stage to launch Bing, although the company is yet to confirm or deny that claim.
But that method is not perfect, according to Agarwal. People might have been presented with options that were all false, then picked the option that was the least false, she says. In an effort to make ChatGPT more reliable, the company has been focusing on cleaning up its dataset and removing examples where the model has had a preference for things that are false.
Jailbreaking ChatGPT: Since ChatGPT’s release, people have been trying to “jailbreak” it, which means finding workarounds to prompt the model to break its own rules and generate racist or conspiratory stuff. This work has not gone unnoticed at OpenAI HQ. Agarwal says OpenAI has gone through its entire database and selected the prompts that have led to unwanted content in order to improve the model and stop it from repeating these generations.
OpenAI wants to listen: The company has said it will start gathering more feedback from the public to shape its models. OpenAI is exploring using surveys or setting up citizens assemblies to discuss what content should be completely banned, says Lama Ahmad. “In the context of art, for example, nudity may not be something that's considered vulgar, but how do you think about that in the context of ChatGPT in the classroom,” she says.
Consensus project: OpenAI has traditionally used human feedback from data labellers, but recognizes that the people it hires to do that work are not representative of the wider world, says Agarwal. The company wants to expand the viewpoints and the perspectives that are represented in these models. To that end, it’s working on a more experimental project dubbed the “consensus project,” where OpenAI researchers are looking at the extent to which people agree or disagree across different things the AI model has generated. People might feel more strongly about answers to questions such as “are taxes good” versus “is the sky blue,” for example, Agarwal says.
✓ A customized chatbot is coming: Ultimately, OpenAI believes it might be able to train AI models to represent different perspectives and worldviews. So instead of a one-size-fits-all ChatGPT, people might be able to use it to generate answers that align with their own politics. “That's where we're aspiring to go to, but it's going to be a long, difficult journey to get there because we realize how challenging this domain is,” says Agarwal.
Here’s my two cents: It’s a good sign that OpenAI is planning to invite public participation in determining where ChatGPT’s red lines might be. A bunch of engineers in San Francisco can’t, and frankly shouldn’t, determine what is acceptable for a tool used by millions of people around the world in very different cultures and political contexts. I’ll be very interested in seeing how far they will be willing to take this political customization. Will OpenAI be okay with a chatbot that generates content that represents extreme political ideologies? Meta has faced harsh criticism after allowing the incitement of genocide in Myanmar on its platform, and increasingly, OpenAI is dabbling in the same murky pond. Sooner or later, it’s going to realize how enormously complex and messy the world of content moderation is.
Deeper Learning
AI is dreaming up drugs that no one has ever seen. Now we’ve got to see if they work.
Hundreds of startups are exploring the use of machine learning in the pharmaceutical industry. The first drugs designed with the help of AI are now in clinical trials, the rigorous tests done on human volunteers to see if a treatment is safe—and really works—before regulators clear them for widespread use.
Why this matters: Today, on average, it takes more than 10 years and billions of dollars to develop a new drug. The vision is to use AI to make drug discovery faster and cheaper. By predicting how potential drugs might behave in the body and discarding dead-end compounds before they leave the computer, machine-learning models can cut down on the need for painstaking lab work. Read more from Will Douglas Heaven here.
Bits and Bytes
The ChatGPT-fueled battle for search is bigger than Microsoft or Google
It’s
not just Big Tech that’s trying to make AI-powered search happen. Will
Douglas Heaven looks at a slew of startups trying to reshape search—for
better or worse. (MIT Technology Review)
A new tool could help artists protect their work from AI art generators
Artists
have been criticizing image making AI systems for stealing their work.
Researchers at the University of Chicago have developed a tool called
Glaze that adds a sort of cloak to images that will stop AI models from
learning a particular artist’s style. This cloak will look invisible to
the human eye, but it will distort the way AI models pick up the image. (The New York Times)
A new African startup wants to build a research lab to lure back talent
This
is cool. South African AI research startup Lelapa wants to convince
Africans working in tech jobs overseas to quit and move back home to
work on problems that serve African businesses and communities. (Wired)
An elite law firm is going to use AI chatbots to draft documents
British
law firm Allen and Overy has announced it is going to use an AI chatbot
called Harvey to help its lawyers draft contracts. Harvey was built
using the same tech as OpenAI’s ChatGPT. The firm’s lawyers have been
warned that they need to fact check any information Harvey generates.
Let’s hope they listen, or this could get messy. (The Financial Times)
Inside the ChatGPT race in China
In
the last week, almost every major Chinese tech company has announced
plans to introduce their own ChatGPT-like products, reports my colleague
Zeyi Yang in his newsletter about Chinese tech. But a Chinese ChatGPT
alternative won’t pop up overnight—even though many companies may want
you to think so." (MIT Technology Review)
Correction: This story has been updated to reflect that Microsoft Bing is not built on ChatGPT, but similar AI language technology customized for search. We apologize for the error.
Stripe Cuts Valuation to $50 Billion After Facing Fundraising HurdlesRead Now
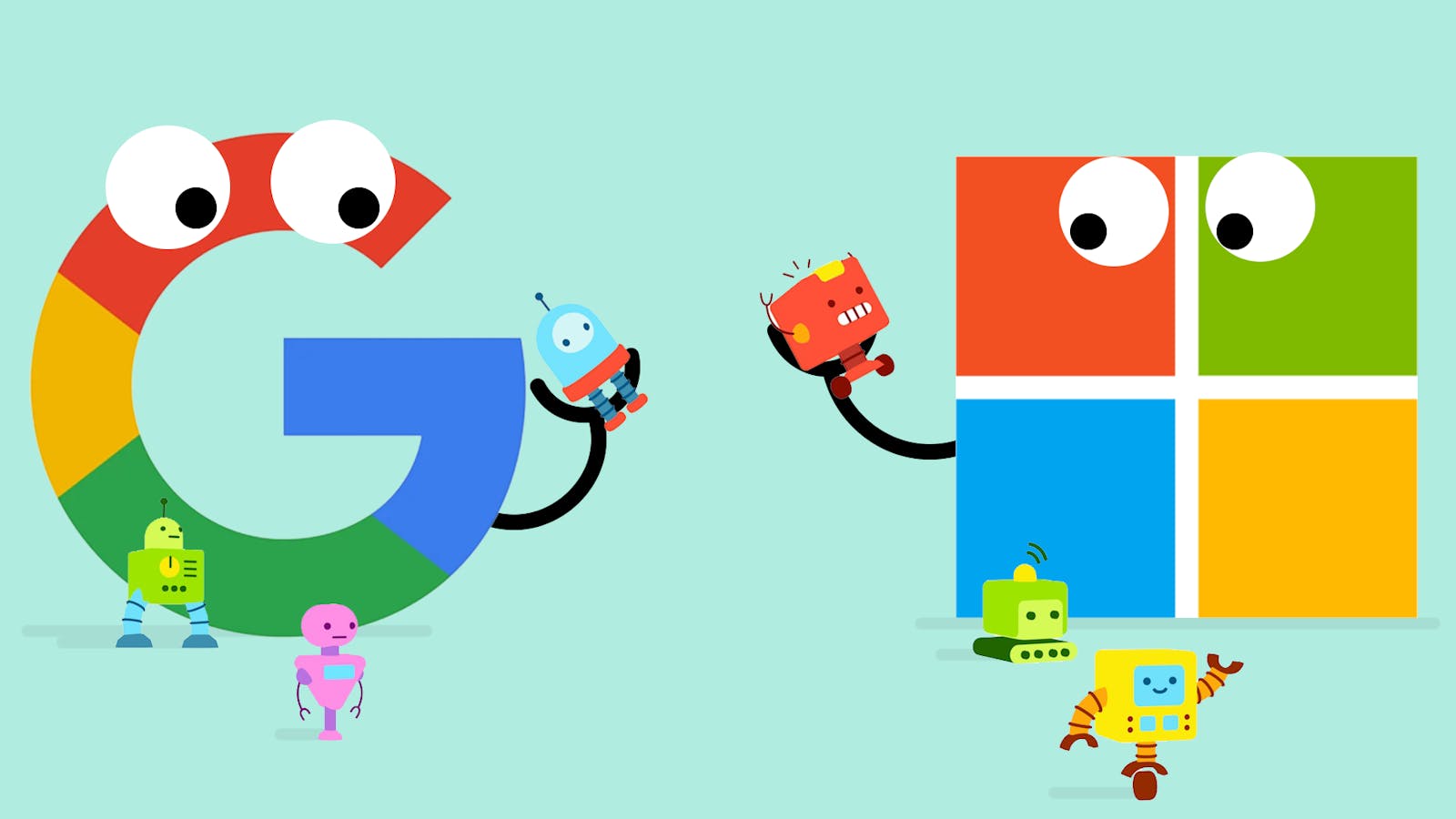
By
| Jan. 9, 2023 6:00 AM PST
Photo: Art by Mike Sullivan via Shutterstock
Microsoft’s big bet on OpenAI, whose ChatGPT software can understand and generate conversational text, is starting to look like a stroke of genius. Microsoft plans to use OpenAI’s tech to improve results in Bing searches and help Word and Outlook customers automatically generate documents and emails using simple prompts, as The Information has reported.
But OpenAI is far from the only startup developing software that can understand language and that larger tech companies such as Google, Amazon, or Meta Platforms may want to license, partner with or acquire. In the last few years, top researchers from OpenAI, Alphabet’s DeepMind and Google, which pioneered the machine-learning techniques used in ChatGPT, have left those companies to launch or join six startups that compete with OpenAI.
Creator Economy startups
At Clubhouse, Many Notable Hires Have Departed
By Kaya Yurieff · March 2, 2023 3:58 PM PST
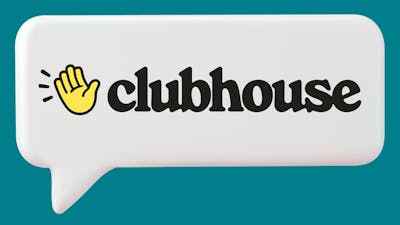
Roughly 20 leaders have departed Clubhouse over the past year or so, including many of the notable hires the social audio app recruited from major companies such as Meta Platforms, Google, TikTok, Spotify and Netflix. All these ex-staffers had vice president, head or lead in their titles or job descriptions (most were heads of something).The social audio app, which turns three years next month,...
Biden’s Cybersecurity Strategy Raises Questions for Software Providers
By Kevin McLaughlin · March 2, 2023
TikTok COO Pappas Blames ‘Xenophobia’ as Congress Moves Closer to Ban
By Kaya Yurieff · March 2, 2023
Everlane CFO To Depart For QVC Parent
By Ann Gehan · March 2, 2023
No comments:
Post a Comment